Understanding Gradient Descent with a simple analogy: The Backbone of Today’s Gen AI
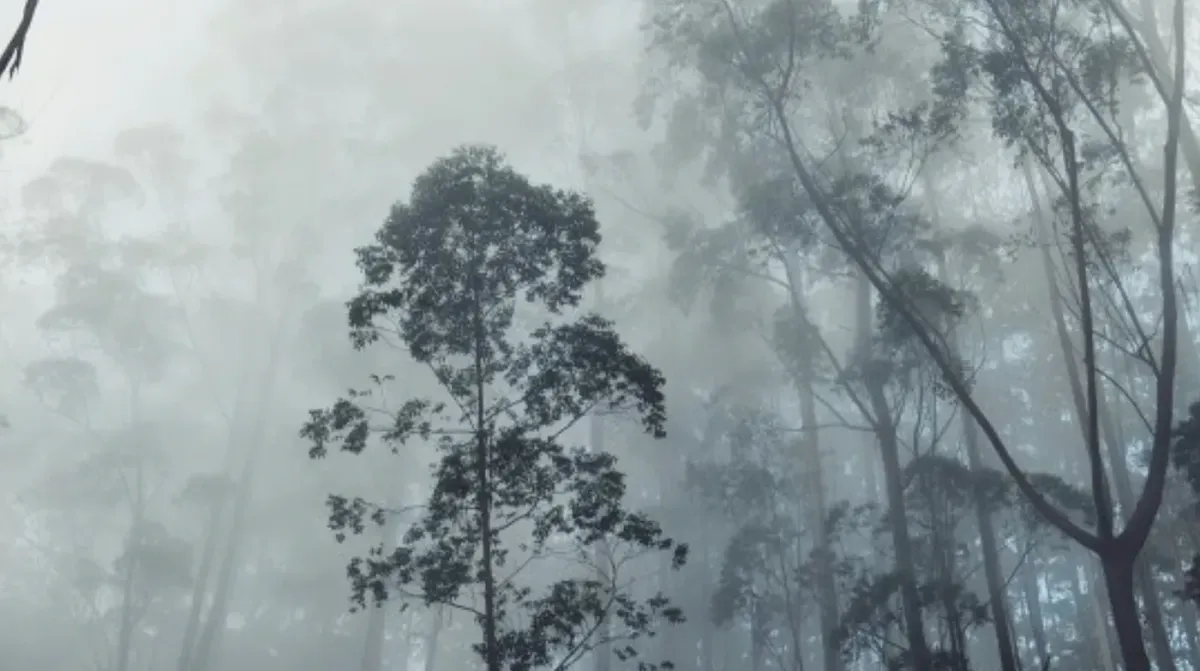
Imagine you’re stuck in thick fog on a mountain and you want to get to the bottom as quickly as possible. You can’t see the path, so you have to rely on feeling the slope of the ground under your feet to find the way down.
You have a special tool that tells you how steep the slope is, but it takes time to use. So, you don’t want to waste too much time measuring the slope, but you still need to make sure you’re heading downhill.
You start by taking a step in any direction and feeling the slope under your feet. Then, you use your tool to check how steep it is. If it’s steep downhill, you take bigger steps in that direction. If it’s steep uphill, you turn around and try another direction.
You keep repeating this process, taking steps and checking the slope, adjusting your direction each time to make sure you’re going downhill as fast as possible.
Eventually, you’ll either reach the bottom of the mountain or get stuck in a hole (like a lake or a valley). But by using this method, you have a good chance of finding your way down efficiently, even though you can’t see the path directly.
In generative AI, there is typically an objective function that quantifies how well the generated data matches the real data. For example, in image generation, this objective function might measure the similarity between the generated images and the real images using metrics like pixel-wise difference or perceptual similarity.
In summary, gradient descent is essential in generative AI for optimizing the parameters of models to generate data that mimics the characteristics of real data. It is a key component in training generative models like GANs, variational autoencoders (VAEs), and other generative models.
#genai #ai #rlhf #learnai
We help SaaS businesses to delight their customers with Ai analytics under a day.